will nexium help gallbladder problems
In a recent study published in on the preprint server Research Square* while it is under review for publication in Scientific Reports, researchers examine the potential use of deep learning (DL) models to diagnose fetal kidney anomalies.
Study: Deep learning prediction of renal anomalies for prenatal ultrasound diagnosis. Image Credit: Tavarius / Shutterstock.com
*Important notice: Research Square publishes preliminary scientific reports that are not peer-reviewed and, therefore, should not be regarded as conclusive, guide clinical practice/health-related behavior, nexium dr 40 mg side effects or treated as established information.
Introduction
DL is a method of machine learning that uses artificial neural networks to rapidly and deeply analyze large datasets to identify key features that may contribute to a given outcome. The immense amounts of data processed using DL could provide new approaches that enhance or change the way healthcare is delivered.
A major advantage of DL is the ability to constantly improve the performance of the model by including additional data, especially since the models excel at recognizing patterns. For example, many models have been trained on medical image analyses, especially when based on convolutional neural networks (CNN).
Ultrasound is the most frequently used medical imaging technology. Importantly, the images generated by ultrasound imaging depend on the skill and other characteristics of both the operator and interpreter, thus making clinically useful DL modeling difficult.
Fetal ultrasound imaging is crucial for observing fetal anatomy and the presence of any malformations. Considering the high rate of congenital anomalies of the kidneys and urinary tract (CAKUT) at one in 500 live births, the incorporation of DL into prenatal screening could allow clinicians to diagnose these conditions earlier to improve patient outcomes.
What did the study show?
The researchers of the current study determined the extent to which DL models could detect fetal kidney abnormalities. To this end, a two-class representation of existing images was utilized, along with a model approach to interpret multiple classes.
The applied model was designed to handle multiple labels or varied in the hierarchical clustering of labels. The researchers also used explainable artificial intelligence (XAI) tools, including Grad-CAM and HiResCAM, to visualize and understand why certain images were misclassified and how the model generated predictions.
About 970 ultrasound images were used to train the current study, which consisted of about 650 controls and 320 images of anomalous kidney anatomy. More specifically, about 260 images exhibited unilateral dilation of the urinary tract (UTD), whereas 64 were images of unilateral multicystic dysplastic kidney (MCDK).
The highest performance of the model was determined by an accuracy exceeding 80%, with a sensitivity of 81% and specificity of 82% using a test dataset. When three classes were used for prediction rather than two, the performance dropped sharply, with a mean accuracy of 72%, sensitivity of about 40%, and specificity of 86%. This could be due to the small number of UTD and MCKD cases, thus making the two-class problem easier to solve for the trained model than the three-class representation.
The two-class model detected 85% of UTD cases as compared to 67% of MCKD cases. The model was also able to characterize clinically relevant features of the image, with HiResCAM more informative than GradCAM; however, the model misclassified images in four cases.
DL was also used to discriminate normal and abnormal conditions in a two-class schema. The best model showed great promise in its generalizability to ultrasound imaging of CAKUT.
The more frequently represented UTD cases were better predicted than the rarer MCKD images. This indicates that providing more cases in the training dataset allowed the model to identify relevant patterns and ultimately distinguish these patterns to a greater degree from normal ultrasound images.
The study findings advance DL-based CAKUT recognition through ultrasound imaging by using, for the first time on such a large scale, an algorithm trained from the beginning. The model also exploits the potential of XAI techniques to improve the interpretation of the model’s predictions.
The potential for misclassification also exists for various reasons. This emphasizes the need to advance the current understanding of DL models to improve their reliability in areas where wrong predictions could be disastrous for the patient.
What are the implications?
Our findings emphasize the potential of deep learning models in predicting kidney anomalies from limited prenatal ultrasound imagery.”
The study findings emphasize the importance for future prospective studies to use standardized fetal data to maximize data quality and representativeness. AI expertise is key to better understand the limitations of current approaches to training machine learning models in fetal ultrasound diagnoses of congenital anomalies. These types of initiatives will facilitate the development of more reliable and accurate AI-based tools for this purpose.
*Important notice: Research Square publishes preliminary scientific reports that are not peer-reviewed and, therefore, should not be regarded as conclusive, guide clinical practice/health-related behavior, or treated as established information.
- Preliminary scientific report. Miguel, O. X., Kaczmarek, E., Lee, I., et al. (2023). Deep learning prediction of renal anomalies for prenatal ultrasound diagnosis. Research Square. doi:10.21203/rs.3.rs-3101390/v1. https://www.researchsquare.com/article/rs-3101390/v1.
Posted in: Child Health News | Device / Technology News | Medical Science News | Medical Research News | Medical Condition News
Tags: Anatomy, Artificial Intelligence, Deep Learning, Healthcare, Imaging, In Utero, Kidney, Machine Learning, Medical Imaging, Prenatal, Research, Technology, Ultrasound
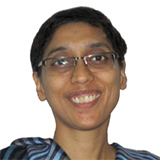
Written by
Dr. Liji Thomas
Dr. Liji Thomas is an OB-GYN, who graduated from the Government Medical College, University of Calicut, Kerala, in 2001. Liji practiced as a full-time consultant in obstetrics/gynecology in a private hospital for a few years following her graduation. She has counseled hundreds of patients facing issues from pregnancy-related problems and infertility, and has been in charge of over 2,000 deliveries, striving always to achieve a normal delivery rather than operative.